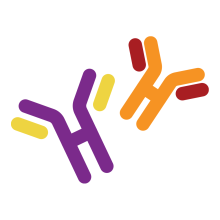
Article
Scaling antibody therapy development with new gene synthesis technology
Monoclonal antibodies have proven themselves to be a safe and effective treatment for a range of diseases, from cancer to infectious and neurodegenerative diseases.1 With over a thousand monoclonal antibody therapies either in clinical use or being trialed, they are now the fastest-growing class of biotherapeutics. This has led to huge growth in the market for monoclonal antibody therapies, which is currently valued at $235 billion as of 2023 but is estimated to rise steeply to $824 billion in the next decade.2
Approximately half of the almost 200 antibodies either approved or in regulatory approval are therapies for cancer indications,1 and many antibody therapies have been developed as research into cancer checkpoints and inhibitors has uncovered a range of antigens suitable for targeting with monoclonal antibodies. Antibody therapy growth is also driven by a range of other therapy areas; one of the biggest drivers is infectious disease therapy, with seven monoclonal antibody therapies for COVID-19 having been authorized for use in the US by the start of 2022. Other indications include immune disorders, cardiovascular disorders, and neurological disorders.
The growth and success of monoclonal antibodies as a therapeutic class has been made possible by extensive progress in antibody engineering, resulting in more effective drugs with fewer side effects. Despite this, a report from 2021 showed that antibody drug discovery processes, including screening and characterization, were considered to be the biggest challenges facing therapeutic antibody research and development.3 The continued rapid growth of the therapeutic antibody market requires tools for antibody discovery and optimization at scale – a challenge that requires a step change in the availability of DNA synthesis.
Using engineering to optimize antibodies for clinical use
Important advances in antibody engineering have enhanced the safety and efficacy of therapeutic antibodies, and are paving the way for the next generation of new and improved antibody drugs.4 Clinical use of monoclonal antibodies requires them to have several properties that ensure their efficacy, safety and ease of use.5 For example, for a drug to be considered for therapeutic use, it generally needs to have a binding affinity of 1nM or less for the target antigen.4 By engineering antibodies using techniques such as site-directed mutagenesis, various properties of therapeutic antibody candidates can be improved with the purpose of enhancing their antigen-binding affinity and specificity, biological efficacy, pharmacokinetics and immunogenicity.6
Several processes are involved in antibody optimization before therapeutic use. Humanization is a process that grafts non-human antigen-binding sequences to human constant regions to reduce immunogenicity and therefore improve the safety of the antibody. Immunogenicity may be further reduced through deimmunization, which aims to remove epitopes predicted to be antigenic. Efficacy is improved through affinity maturation using methods such as targeted and random mutagenesis and in silico approaches, which aim to improve the strength and specificity of binding of the antibody to its antigen. Further optimization is aimed at improving pharmacokinetics, thermostability and stability, among other factors. Many mutations may be combined to develop an antibody with the desired properties through iterative design.7
Once modifications have been identified using a range of tools that includes targeted and random mutagenesis, chain shuffling and AI design tools, experimental testing and characterization are required to functionally test the antibodies for the desired properties and guide further modification - a time and resource-demanding process. Although machine learning is emerging as a tool able to significantly speed up parts of this process by predicting effective changes, there is still a large burden that comes from testing optimized antibody variants, particularly as the chance of finding an antibody with the desired profile increases with the number of variants that can be tested.8 Consequently, the time and costs associated with lead-candidate optimization can often take up the majority of the pre-clinical discovery and development cycle.6
Improving DNA synthesis to reduce timeframes
To identify the most promising monoclonal antibodies for drug development, pharmaceutical companies use protocols that screen thousands or tens-of-thousands of antibodies per screening cycle based on basic features of target binding. Rescue of the coding sequences from these primary binding antibodies provides the starting point for an optimization process designed to develop the most effective therapeutic antibody sequence. Lab automation and machine learning has been transformative in scaling up antibody functional screens and guiding engineering to improve the quality of candidates; however, significant challenges remain in DNA availability, ultimately limiting the number of antibodies that can enter these optimization phases.
To characterize each sequence, DNA needs to be synthesized, cloned and transformed and colonies picked for sequencing to find error-free sequences, before being expressed for functional characterization. There are two major bottlenecks in this process: the time to receive synthesized DNA and dealing with errors in the DNA synthesis process.
Currently, a significant amount of antibody development pipeline is taken up designing and waiting for DNA. Due to the resources and labor required, DNA synthesis and assembly have become centralized services, provided by a handful of reagent manufacturers. Consequently, DNA synthesis and delivery may take as much as 25% of the total time needed for functional screening of antibody variants.
Errors incorporated during DNA synthesis also present an additional challenge, owing to the additional post-synthesis steps required to identify error-free sequences. A significant number of the steps involved in antibody functional screens – cloning, transformation, colony picking and sequencing – are made necessary by the need to identify error-free sequences amongst erroneous ones.
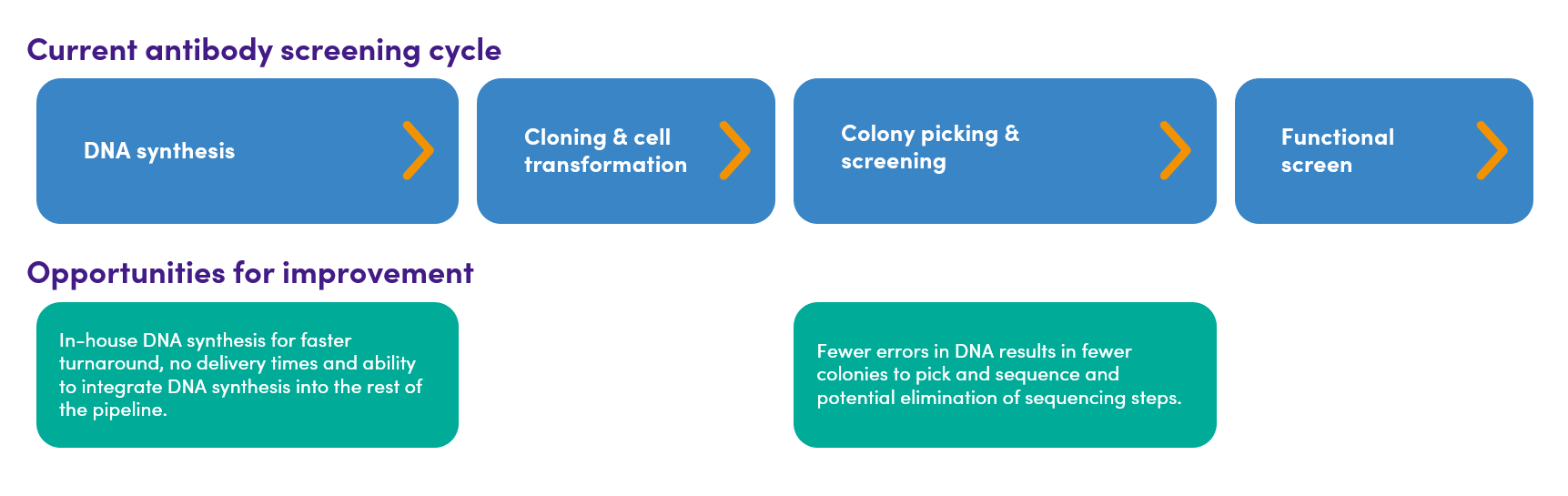
Developments in DNA synthesis technology present opportunities to resolve these two bottlenecks. Evonetix is developing technology that reinvents DNA synthesis, putting sophisticated, accurate gene synthesis capabilities into a benchtop device, thus enabling the synthesis of long DNA on-demand and removing the need to wait for delivery. In addition, benchtop DNA synthesis will more easily integrate into existing antibody development pipelines, reducing manual steps and further accelerating screening.
Evonetix’s patented Binary Assembly® process and thermal control allow oligonucleotides to be automatically combined and error-containing sequences removed. This significantly improves the quality of DNA synthesized and reduces post-synthesis steps by reducing the number of colonies that need to be analyzed and potentially entirely removing the need for sequencing.
Outlook
Antibody therapies are a large and growing class of therapeutics that hold much promise in the areas of cancer, immune diseases and many more. However, accelerated growth in this area requires streamlining of the antibody development process. Improved gene synthesis capabilities, alongside breakthroughs in machine learning and automation are poised to propel antibody therapeutics to even greater heights.